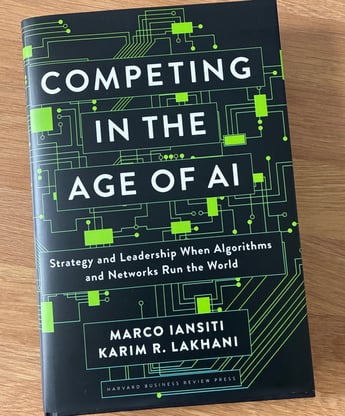
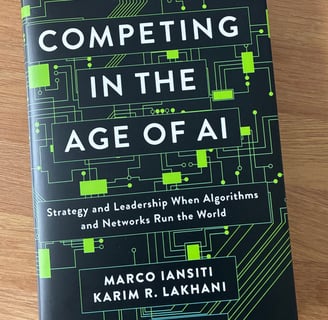
Competing in the Age of AI
Marco Iansiti, Karim R. Lakhani, 2020.
Link to book on Amazon
Competing in the Age of AI is a seminal work that argues AI is not just a tool but a fundamental force redefining the nature of firms and competition. Iansiti and Lakhani, both Harvard Business School professors, draw on extensive research and real-world examples—such as Amazon, Microsoft, Airbnb, and Ant Financial—to illustrate how AI-centric organizations leverage data, analytics, and algorithms to overcome traditional constraints on growth. The book’s core premise is that AI enables a new operating architecture, transforming how companies create, capture, and deliver value. It’s a compelling read for executives, entrepreneurs, and strategists, though it occasionally assumes familiarity with technical concepts and focuses predominantly on tech giants, which may limit its applicability for smaller firms.
The book is structured into eight chapters, each tackling a facet of AI’s impact on business. It balances theoretical frameworks with practical insights, making it accessible yet rigorous. Strengths include its forward-looking analysis, vivid case studies, and emphasis on strategic adaptation. Weaknesses include a lack of clear distinction between AI and related terms like machine learning, and limited guidance for smaller organizations. Despite these, it’s a must-read for understanding the AI-driven economy, earning accolades like being named a “Top Ten Technology Book of 2020” by Forbes.
Competing in the Age of AI Review by chapter
Chapter 1: The Age of AI
Summary: This chapter introduces AI as a transformative force, not just a technology but a new foundation for business operations. It highlights how AI-driven firms like Amazon and Google use data and algorithms to redefine competition, moving beyond human-centric models to scalable, automated systems.
Key Points:
AI is the “universal engine of execution,” integrating data and algorithms to drive decisions.
Traditional firms face disruption from AI-centric models that prioritize speed and scale.
Digital operating models enable continuous learning, unlike static traditional processes.
Case studies (e.g., Ant Financial) show AI’s ability to serve millions with minimal human intervention.
Application: Businesses should assess their reliance on manual processes and identify areas where AI can enhance efficiency, such as customer service or supply chain management. For example, a retail company could implement AI-driven demand forecasting to optimize inventory, reducing costs and improving responsiveness. Starting with pilot projects in high-impact areas can build momentum for broader transformation, ensuring alignment with strategic goals.
Chapter 2: Rethinking the Firm
Summary: The chapter redefines the firm as a data-driven entity, where AI removes constraints on scale, scope, and learning. It contrasts traditional firms, limited by human capacity, with AI-driven ones that leverage networks and analytics for exponential growth.
Key Points:
AI enables firms to scale without proportional increases in resources.
Scope expands as AI connects diverse industries (e.g., Amazon’s move into healthcare).
Learning effects amplify as more data improves algorithms, creating a virtuous cycle.
Traditional organizational silos hinder AI adoption; integration is critical.
Application: Companies should re-evaluate their organizational structure to break down silos and centralize data access, enabling AI to drive cross-functional insights. For instance, a manufacturing firm could integrate IoT sensors with AI analytics to predict equipment failures, enhancing efficiency across production lines. This requires investing in data infrastructure and fostering a culture of collaboration to maximize AI’s potential.
Chapter 3: The AI Factory
Summary: This chapter introduces the “AI factory” concept—a scalable decision-making engine powered by data, software, and algorithms. It explains how firms like Google and Microsoft build AI factories to systematize innovation and execution.
Key Points:
AI factories standardize processes, enabling rapid experimentation and deployment.
Data pipelines are the backbone, feeding algorithms with real-time insights.
Governance ensures ethical AI use and alignment with business objectives.
Example: Microsoft’s Azure platform leverages AI factories for global scalability.
Application: Organizations can adopt the AI factory model by creating centralized data platforms that support modular AI applications. A financial services firm, for example, could develop an AI factory to automate fraud detection, using real-time transaction data to refine models. This requires investing in cloud infrastructure and training teams to manage AI workflows, ensuring scalability and adaptability.
Chapter 4: Rearchitecting the Firm
Summary: Transitioning to an AI-centric model requires redesigning the firm’s architecture—processes, culture, and technology. The chapter uses Amazon’s transformation to illustrate how legacy systems must evolve to support AI-driven operations.
Key Points:
AI demands a shift from rigid hierarchies to flexible, data-driven structures.
Legacy systems often lack the agility needed for AI integration.
Cultural change is essential to embrace experimentation and failure.
Amazon’s use of APIs and microservices exemplifies a modular architecture.
Application: Firms should audit their technology stack and processes to identify barriers to AI adoption, then prioritize modular designs that support rapid iteration. A healthcare provider could rearchitect its patient management system to integrate AI diagnostics, using APIs to connect disparate data sources. Leaders must champion cultural shifts, encouraging teams to experiment and learn from AI-driven insights.
Chapter 5: Becoming an AI Company
Summary: This chapter outlines practical steps for traditional firms to become AI-driven, emphasizing gradual transformation. It highlights cases like Novartis, which used AI to streamline drug development.
Key Points:
Start with pilot projects to test AI’s impact on specific functions.
Build data capabilities to ensure quality and accessibility.
Reskill employees to work alongside AI, focusing on complementary skills.
Leadership commitment is crucial to sustain transformation.
Application: Traditional businesses should launch small-scale AI initiatives in areas with clear ROI, such as marketing personalization or predictive maintenance. A logistics company could pilot AI route optimization to reduce fuel costs, then scale successful models. Investing in employee training and securing executive buy-in ensures long-term success, aligning AI with strategic priorities.
Chapter 6: Strategy for a New Age
Summary: AI reshapes strategy by prioritizing networks over industries. The chapter discusses concepts like multihoming, network bridging, and disintermediation, using Uber and Alibaba as examples.
Key Points:
Network effects drive value creation in AI-driven firms.
Multihoming weakens platforms if users can easily switch (e.g., Uber vs. Lyft).
Network bridging connects disparate ecosystems for competitive advantage.
Strategy shifts from industry analysis to network dynamics.
Application: Strategists should map their firm’s network ecosystem to identify opportunities for bridging or strengthening ties. A retail platform could use AI to connect suppliers and customers across regions, enhancing value through personalized recommendations. Monitoring multihoming risks ensures retention, while leveraging data-driven insights redefines competitive positioning.
Chapter 7: Strategic Collisions
Summary: This chapter explores “collisions” between AI-driven and traditional firms, showing how digital models disrupt industries. Nokia’s decline versus Apple’s rise illustrates the stakes of failing to adapt.
Key Points:
AI-driven firms outpace traditional ones through scale and learning advantages.
Collisions reshape industries, forcing legacy firms to innovate or decline.
Nokia’s focus on hardware ignored software-driven ecosystems.
Adaptation requires rethinking value creation and delivery.
Application: Legacy firms must proactively adopt AI to avoid disruption, focusing on areas where digital models threaten their core. A traditional retailer could integrate AI-driven e-commerce to compete with Amazon, using analytics to enhance customer experiences. Regular competitive analysis and willingness to pivot ensure resilience against digital disruptors.
Chapter 8: The Ethics of Digital Scale, Scope, and Learning
Summary: The final chapter addresses ethical challenges of AI, such as bias, privacy, and job displacement. It calls for responsible leadership to balance innovation with societal impact.
Key Points:
AI’s scale amplifies ethical risks, like biased algorithms.
Privacy concerns arise from extensive data collection.
Job displacement requires reskilling to mitigate social harm.
Leaders must prioritize transparency and accountability.
Application: Companies should establish AI ethics frameworks, incorporating regular audits to detect bias and ensure compliance with privacy regulations. A tech firm could implement transparent AI policies and reskilling programs to support employees displaced by automation. Engaging stakeholders and aligning AI initiatives with societal values builds trust and sustainability.
Overall Critique and Recommendation
Competing in the Age of AI is a thought-provoking guide for navigating the AI-driven economy. Its strengths lie in its clear framework, rich examples, and emphasis on actionable strategies. However, it could better address AI’s accessibility for smaller firms and clarify technical distinctions (e.g., AI vs. machine learning). The book’s focus on tech giants occasionally overshadows broader applicability, and some chapters feel repetitive. Nonetheless, it’s an essential resource for leaders seeking to harness AI’s potential.
Recommendation: Ideal for executives, strategists, and entrepreneurs in medium-to-large organizations, particularly those in tech-heavy industries. Smaller firms may need to adapt its insights creatively. Pair it with practical AI implementation guides for a balanced approach.
BLAGDEN DIGITAL LIMITED
Expert guidance for your digital transformation journey.
contact@blagdendigital.com
© 2025. All rights reserved.